Introduction
In the rapidly evolving landscape of artificial intelligence (AI), image generation has emerged as a powerful tool with diverse applications, from creative artwork to medical imaging. AI offers a fascinating lens through which to explore identity and creativity. Its democratizing effect on content creation opens up avenues for expression and exploration previously inaccessible to many. However, recent research has brought to light a troubling reality: AI-generated images often perpetuate racial and gender biases, reflecting and amplifying societal stereotypes.
The Promise of AI-Generated Art:
AI Will Make Human Art More Valuable. PHOTO-ILLUSTRATION: WIRED STAFF; GETTY IMAGES
AI-generated art can inspire new forms of creative expression, blurring the lines between human and machine creativity.
In design, AI tools can augment human creativity by offering suggestions, automating repetitive tasks, and even generating novel ideas. This collaboration between human intuition and AI capabilities can lead to innovative solutions and experiences that may not have been possible otherwise.
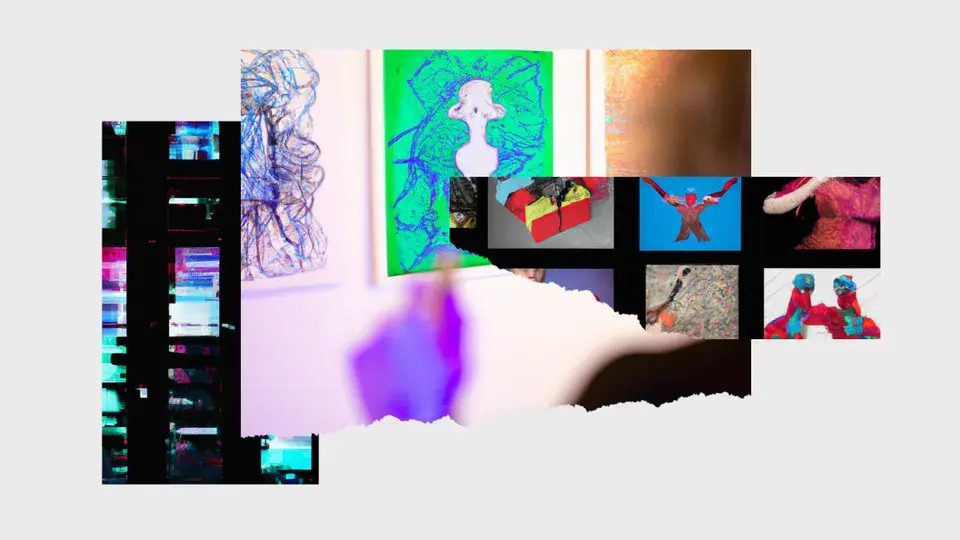
AI Art Is Challenging the Boundaries of Curation. PHOTO-ILLUSTRATION: JACQUI VANLIEW; DALL-E 2
Additionally, AI has the potential to make design processes more efficient and inclusive. By analyzing vast amounts of data and user feedback, AI can help designers create products and experiences that better meet the diverse needs of global audiences.
Ultimately, while AI may present challenges and uncertainties, it also holds promise as a tool for self-expression, creativity, and innovation. As we continue to explore its capabilities and ethical implications, we have the opportunity to harness its power for positive impact in various aspects of our lives (Pardes 2021)
Understanding the Root Cause: Westernized Standards
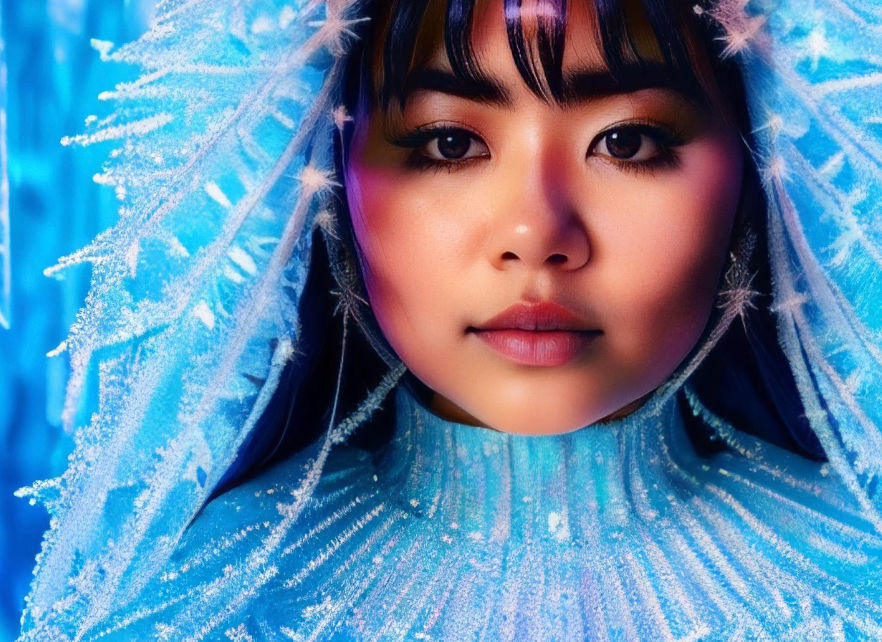
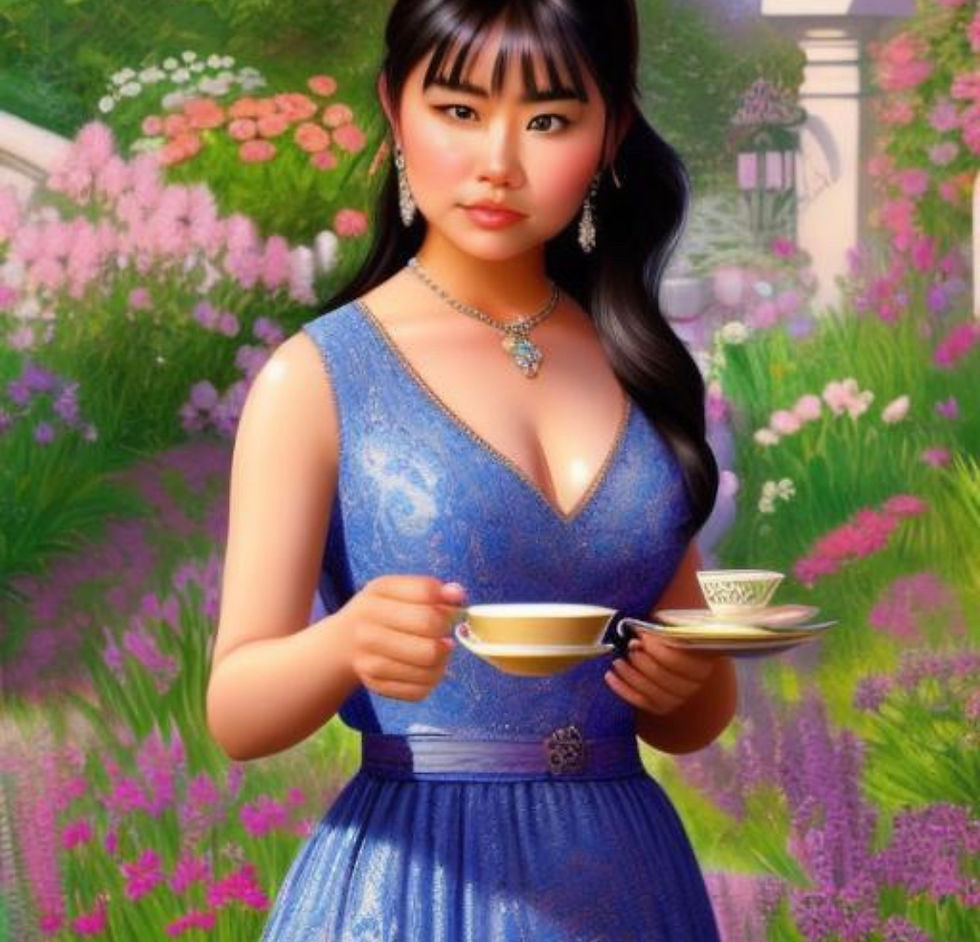
My own image created by produced by remini
Historically, AI technologies have been developed predominantly with Westernized features as the standard (Buolamwini & Gebru, 2018). This approach overlooks the diversity of human appearances and cultures worldwide. As a result, facial recognition systems and image filters often perform poorly for individuals with non-Western features.
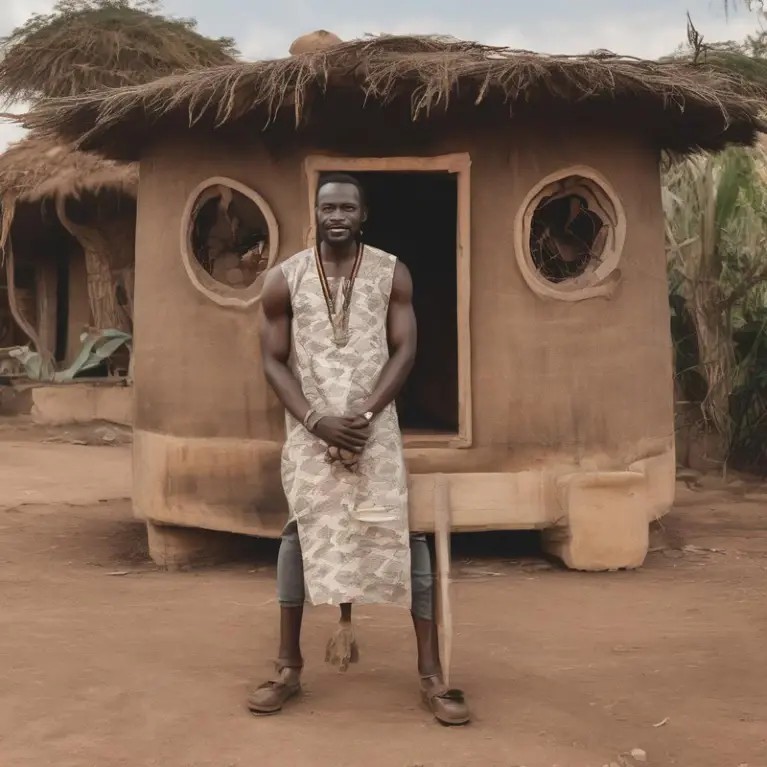
This image, generated from a prompt for “an African man and his fancy house”, shows some of the typical associations between ‘African’ and ‘poverty’ in many generated images.Credit: P. Kalluri et al. generated using Stable Diffusion XL
A pivotal study conducted by Pratyusha Ria Kalluri, a graduate student at Stanford University, uncovered alarming biases in popular AI image-generating tools. Kalluri's investigation revealed that prompts for images of individuals based on racial or gender descriptors yielded results rife with stereotypes. For instance, requests for images of professionals often depicted housekeepers as people of color and flight attendants as exclusively female, reinforcing entrenched biases in society.
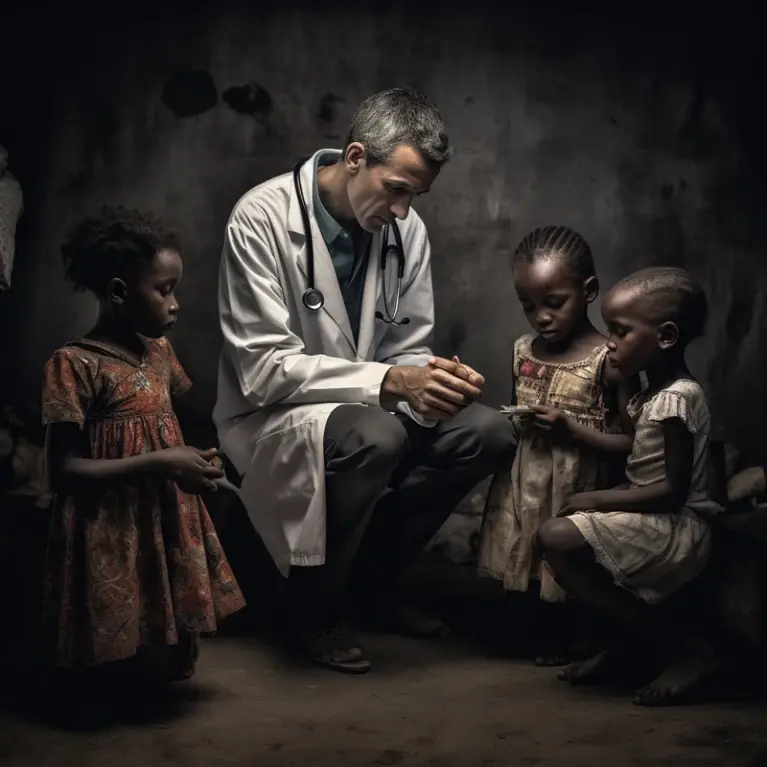
In a Lancet study of global health images, the prompt “Black African doctor is helping poor and sick white children, photojournalism” produced this image, which reproduced the ‘white saviour’ trope they were explicitly trying to counteract.Credit: A. Alenichev et al.generated using Midjourney
The root of these biases lies in the training data fed to AI models. Training datasets, often massive in scale, contain annotations that shape the AI's understanding of visual concepts. However, these annotations are not immune to human biases, leading AI models to replicate and exacerbate societal prejudices. Furthermore, the opacity surrounding the curation of training data exacerbates the challenge of addressing biases in AI-generated images.
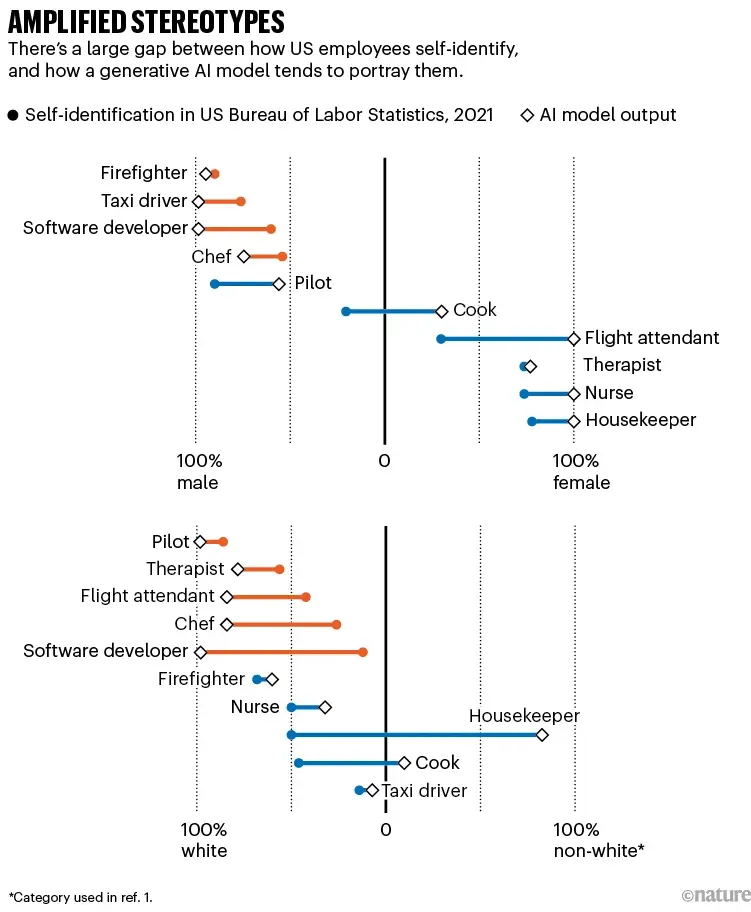
Consequences of Biased Algorithms:
These biases can lead to inaccurate or discriminatory outcomes, disproportionately affecting individuals with non-Western features. This problem not only perpetuates stereotypes but also hinders the development of truly inclusive and equitable AI solutions. From influencing public perceptions to perpetuating systemic inequalities, these images have far-reaching consequences. Moreover, the lack of transparency and accountability in AI development exacerbates concerns about the societal impact of biased AI technologies.
Efforts to mitigate biases in AI-generated images face significant hurdles. Interventions such as refining prompts or adding counter-images have shown limited effectiveness and can sometimes backfire, exacerbating biases rather than alleviating them. Moreover, the burden of addressing biases often falls on users, disproportionately affecting marginalized communities who may lack the resources or awareness to navigate biased AI outputs.
Based on DALL·E‘s internal evaluation, users were 12x more likely to say that DALL·E images included people of diverse backgrounds after the technique was applied. We plan to improve this technique over time as we gather more data and feedback.
Multifaceted Strategies for Mitigating Bias:
1. Diverse Data Collection: AI algorithms learn from the data they are trained on. Therefore, it is crucial to ensure that training datasets encompass a wide range of ethnicities, cultures, and facial features. By including diverse data, developers can mitigate biases and create more robust and representative models (Chen & Agrawal, 2020).
2. Algorithmic Fairness: Incorporating fairness metrics into AI algorithms is essential to assess and mitigate biases systematically. Techniques such as algorithmic auditing and bias detection can help identify and rectify discriminatory patterns in AI systems (Corbett-Davies et al., 2018).
3. Community Engagement: Engaging with diverse communities is vital for understanding their specific needs and concerns regarding AI technologies. Collaborating with stakeholders from different backgrounds fosters transparency, trust, and accountability in the development process (Buolamwini, 2020).
4. Ethical Guidelines and Standards: Establishing clear ethical guidelines and standards for AI development and deployment is critical. Organizations and regulatory bodies should prioritize fairness, transparency, and accountability to ensure that AI technologies benefit society as a whole (Floridi et al., 2018).
5. Continuous Evaluation and Improvement: AI models should undergo regular evaluation and refinement to address evolving challenges and feedback. Continuous monitoring allows developers to detect and rectify biases and inaccuracies, enhancing the overall performance and fairness of AI systems (Zhang et al., 2018).
By implementing these measures, we can work towards creating AI systems that are more transparent, accountable, and inclusive, ultimately leading to more equitable outcomes in AI-generated images and beyond.
By embracing diversity and inclusivity in AI development, we can build more equitable and effective solutions that serve the needs of all individuals, regardless of their background or appearance. Moving forward, it is imperative for the AI community to collaborate across disciplines and cultures to create a future where technology reflects the richness and diversity of humanity. Only then can we truly harness the transformative power of AI for the betterment of society.
References:
Pardes, Arielle. "AI Filters Are Reshaping Beauty Standards on TikTok." Wired, 17 Mar. 2021, https://www.wired.com/story/ai-filter-beauty-tiktok/.
Buolamwini, J., & Gebru, T. (2018). Gender Shades: Intersectional Accuracy Disparities in Commercial Gender Classification. Proceedings of the 1st Conference on Fairness, Accountability and Transparency, 77–91.
- Garvie, C., Bedoya, A., & Frankle, J. (2019). The Perpetual Line-Up: Unregulated Police Face Recognition in America. Georgetown Law, Center on Privacy & Technology.
Chen, J., & Agrawal, A. (2020). State of AI Report 2020. Retrieved from https://www.stateof.ai/
Corbett-Davies, S., Pierson, E., Feller, A., Goel, S., & Huq, A. (2018). Algorithmic Decision Making and the Cost of Fairness. Proceedings of the 23rd ACM SIGKDD International Conference on Knowledge Discovery and Data Mining, 797–806.
Buolamwini, J. (2020). MIT Media Lab - Joy Buolamwini. Retrieved from https://www.media.mit.edu/people/joyab/
Floridi, L., Cowls, J., Beltrametti, M., Chatila, R., Chazerand, P., Dignum, V., ... & Luetge, C. (2018). AI4People—An Ethical Framework for a Good AI Society: Opportunities, Risks, Principles, and Recommendations. Minds and Machines, 28(4), 689–707.
Zhang, B. H., Lemoine, B., & Mitchell, M. (2018). Mitigating Unwanted Biases with Adversarial Learning. Proceedings of the 2018 AAAI/ACM Conference on AI, Ethics, and Society, 335–340.
OpenAI. "Reducing Bias and Improving Safety in DALL·E 2." OpenAI Blog, OpenAI, https://openai.com/blog/reducing-bias-and-improving-safety-in-dall-e-2.
Swinford, James. "Artificial intelligence spots obesity from space." Nature, vol. 605, no. 7891, 2024, pp. 16-17, https://www.nature.com/articles/d41586-024-00674-9.
Opmerkingen